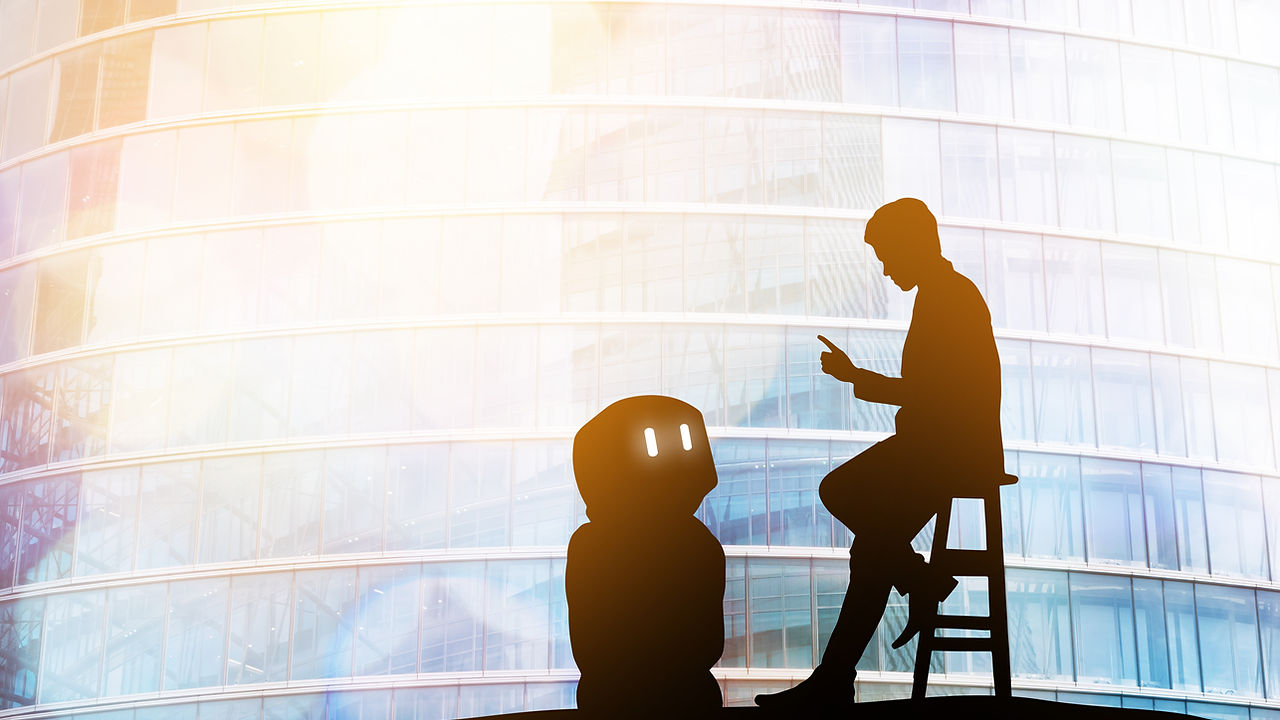
IEEE IRC 2021
Keynotes

​
Stanford University, USA
​
Title: Joint Learning for 3D Perception and Action
​
Abstract: Many challenges remain in applying machine learning to robotic perception in settings where obtaining massive annotated data is difficult. We discuss approaches that aim to reduce the supervision load for learning algorithms in the visual and geometric domains by leveraging correlations among data as well as among learning tasks -- what we call joint learning. The basic notion is that inference problems do not occur in isolation but rather in a "social context" that can be exploited to provide self-supervision by enforcing consistency, thus improving performance and increasing sample efficiency. An example is voting mechanisms where multiple "experts" must collaborate on predicting a particular outcome, such as an object detection. This is especially challenging across different modalities, such as when mixing point clouds with image data, or geometry with language data. Another example is the use of cross-task consistency constraints, as in the case of inferring depth and normals from an image, which are obviously correlated. The talk will present a number of examples of joint learning and of methods that facilitate information aggregation, including the above as well as 3D object pose estimation and spatio-temporal data consolidation.
​
Bio: Professor Guibas heads the Geometric Computation group in the Computer Science Department of Stanford University and is a member of the Computer Graphics and Artificial Intelligence Laboratories. He works on algorithms for sensing, modeling, reasoning, rendering, and acting on the physical world. Guibas interests span computational geometry, geometric modeling, computer graphics, computer vision, sensor networks, robotics, and discrete algorithms --- all areas in which he has published and lectured extensively. Current foci of interest include geometric modeling with point cloud data, deformations and contacts, organizing and searching libraries of 3D shapes and images, sensor networks for lightweight distributed estimation / reasoning, analysis of GPS traces and other mobility data, and modeling the shape and motion biological macromolecules and other biological structures. More theoretical work is aimed at investigating fundamental computational issues and limits in geometric computing and modeling.
Stanford University, USA
​
Title: The New Role of Physics Simulation in AI
​
Abstract: Numerical simulation of physical phenomena is a powerful tool embraced by scientists and engineers for decades. In the quest to leverage machine learning for developing AI-enabled robots, physics simulation creates an ideal proving ground for developing intelligent robots that can both learn from their mistakes and be verifiable. In addition, physics simulation can generate a wealth of labeled training data in a short amount of time at a low cost. When it comes to tasks involving physical human-robot interaction, learning from physically simulated humans and environments enables robots to safely learn from failure without putting real people at risk. However, presently the simulation tool is not very effective, as many designs produced in simulation fail to deliver in the real world—the so-called sim-to-reality gap. The sim-to-real problem is further complicated by deploying policies to physically interact with people in the real world.
While most recent work on sim-to-real transfer problems focused on improving control policies, my work show that one can overcome the sim-to-real gap by improving physics simulation processes. In this talk, I will present my recent work on creating "learnable" physics engines along with efficient techniques for training them. I will also report the current progress on sim-to-real transfer with humans in the environment, actively interacting with the robots through physical contacts.
​
Bio: C. Karen Liu is an associate professor in the Computer Science Department at Stanford University. Prior to joining Stanford, Liu was a faculty member at the School of Interactive Computing at Georgia Tech. She received her Ph.D. degree in Computer Science from the University of Washington. Liu's research interests are in computer graphics and robotics, including physics-based animation, character animation, optimal control, reinforcement learning, and computational biomechanics. She developed computational approaches to modeling realistic and natural human movements, learning complex control policies for humanoids and assistive robots, and advancing fundamental numerical simulation and optimal control algorithms. The algorithms and software developed in her lab have fostered interdisciplinary collaboration with researchers in robotics, computer graphics, mechanical engineering, biomechanics, neuroscience, and biology. Liu received a National Science Foundation CAREER Award, an Alfred P. Sloan Fellowship, and was named Young Innovators Under 35 by Technology Review. In 2012, Liu received the ACM SIGGRAPH Significant New Researcher Award for her contribution in the field of computer graphics.
​
