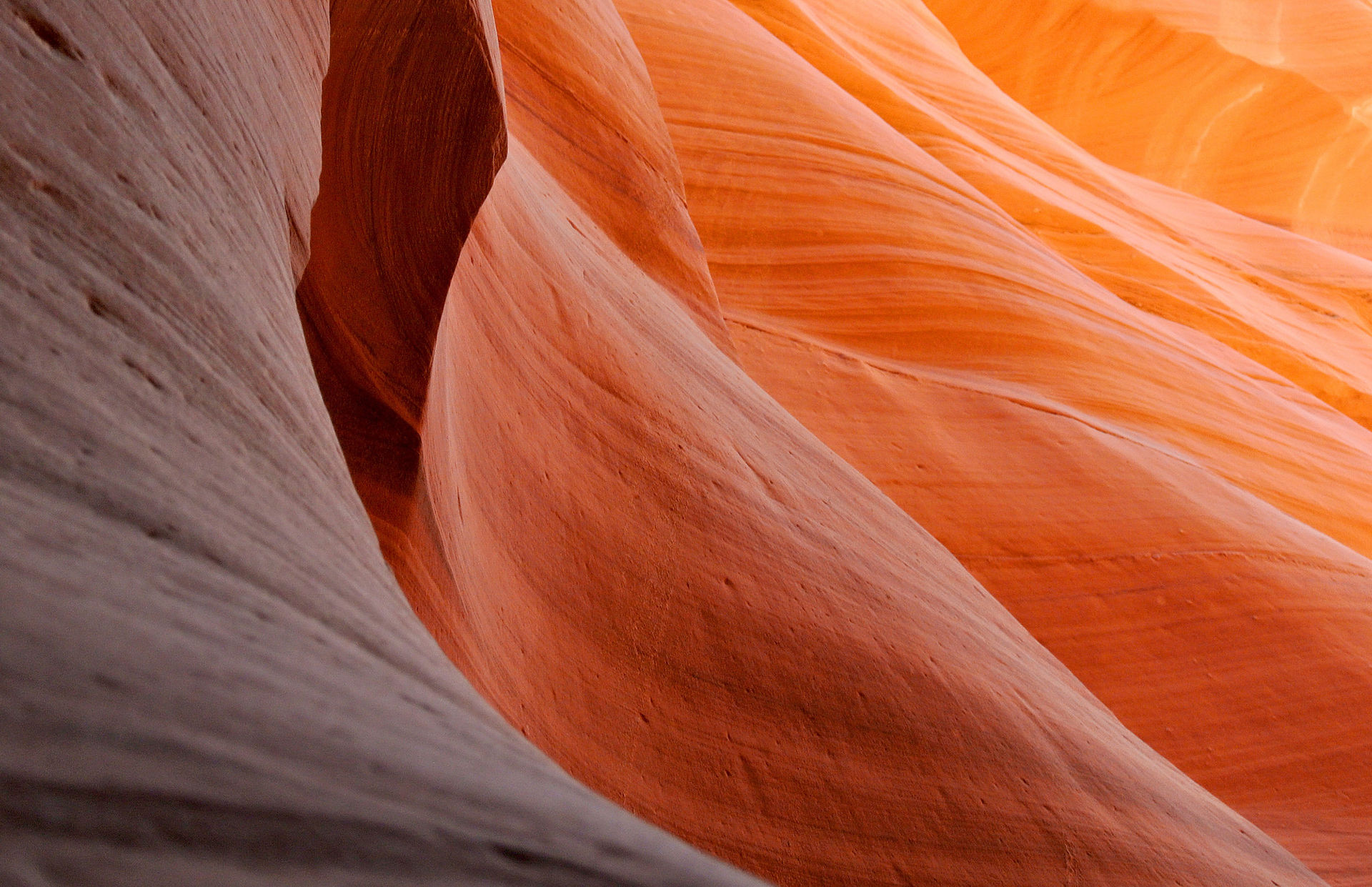

HCCAI 2020, TransAI 2020, ai4i 2020
​
Joint Keynotes
(in alphabetical order)
​
​
Edward Chang
HTC Research & Healthcare (DeepQ)
​
Title: Scarcity, Diversity, and Privacy of Data in Artificial Intelligence for Precision Medicine
Abstract: Recent successes of AI in several application domains attest the importance of big data in achieving high performance in domain-specific metrics. In the healthcare domain, however, big data in the million scale is generally not available. Furthermore, safeguarding data privacy is extremely crucial. This talk first enumerates key data issues in applying AI in the healthcare domain including data scarcity, out of distribution, and data privacy. Then, we present remedies including transfer learning for remedying data scarcity, knowledge-guided multimodal learning for out of distribution generalization, and distributed ledgers for preserving data privacy.
Bio: Edward Chang has acted as the President of AI Research and Healthcare (DeepQ) at HTC since 2012. He also currently serves as an adjunct professor at Stanford University. His most recent notable work is co-leading the DeepQ project to win the XPRIZE medical IoT contest in 2017 with a 1M USD prize. Prior to his current posts, Ed was a director of Google Research from 2006 to 2012, leading research and development in areas including scalable machine learning, indoor localization, and Google Q&A. His contributions in pioneering data-driven machine learning (US patents 8798375 and 9547914) and his ImageNet sponsorship helped fuel the success of AlexNet and the recent resurgence of AI. His developed open-source codes in parallel SVMs, parallel LDA, parallel spectral clustering, and parallel frequent itemset mining (adopted by Berkeley Spark) have been collectively downloaded over 30,000 times. Prior to Google, Ed was a full professor of Electrical & Computer Engineering at the University of California, Santa Barbara. He joined UCSB in 1999 after receiving his PhD from Stanford University. Ed is an IEEE Fellow for his contributions to scalable machine learning.
​
​
Chris Dede
Harvard University
​
Title: AI and New Division of Labor: Deskilling or Upskilling Jobs?
Abstract: Economists describe the "division of labor" as which parts of a task are done by people and which parts by machines. Advances in machine learning are expected to affect most jobs in the coming decade, including "high skill" jobs such as teaching, with computers taking over part of the role. When that occurs, the person can either be deskilled (doing less of the job, and perhaps working for the machine) or upskilled (using the cognitive resources freed by machines doing part of the task to extend job performance by complementing the computer). The choice is AI vs IA (intelligence augmentation).
In measuring success by high-stakes tests, we are preparing students for jobs deskilled by artificial intelligence, which does quite well on these measures. Instead, children should learn what AI cannot do, preparing themselves to roles upskilled in which people working with smart machines accomplish more than either can unaided. To achieve a bright future rather than a dystopian workplace, education at all levels must focus on the knowledge and skills machine learning cannot attain.
Bio: Chris Dede is the Timothy E. Wirth Professor in Learning Technologies at Harvard's Graduate School of Education (HGSE). His fields of scholarship include emerging technologies, policy, and leadership. In 2007, he was honored by Harvard University as an outstanding teacher, and in 2011 he was named a Fellow of the American Educational Research Association. From 2014-2015, he was a Visiting Expert at the National Science Foundation Directorate of Education and Human Resources. His edited books include: Scaling Up Success: Lessons Learned from Technology-based Educational Improvement, Digital Teaching Platforms: Customizing Classroom Learning for Each Student, Teacher Learning in the Digital Age: Online Professional Development in STEM Education, Virtual, Augmented, and Mixed Realities in Education, and Education at Scale: Engineering Online Learning and Teaching.
​
​
​
Sina Fakhraee and Dennis Sawyers
Microsoft, USA
​
Title: Building end-to-end and scalable AI/ML solutions using Azure Machine Learning Platform
​
Abstract: A typical end-to-end machine learning solution involves data preparation (e.g. data ingestion, data pre-processing, feature engineering, etc.), building models (e.g. set of experiments to train and validate models using different algorithms and relevant hyperparameters) and lastly deploying and monitoring trained models. A few main challenges that many data scientists face are how to streamline this process, how to keep track of the model building experiments for reproducibility and collaboration, how to repeat this process (e.g. retrain model with new dataset, redeploy and monitor new models) which is knowns as MLOps and more importantly for production ML systems, how to do all this at scale.
In this talk we will give an overview of Azure Machine Learning (AML) and will discuss how to address the above challenges using AML. In the context of a demo we will show how AML have enabled our customers to build high performing demand forecasting models using many models pattern which can ultimately be deployed for real-time or batch inference.
Bio: Sina Fakhraee has recently joined Microsoft as a Data & AI cloud solution architect. In this role Sina helps his customers in defining, designing and implementing AI/ML solutions at scale on Azure Cloud Platform. These solutions include applying the best practices for data ingestion, feature engineering, model training all the way to model deployment for real time or batch consumption. In some cases, customers may already have this pipeline on-premises and want to move to the cloud which Sina helps in migrating their analytics solutions to the cloud. Prior to Microsoft, Sina worked at Ford Motor Company for 9 years holding a variety of technical lead positions such as senior analytics solution architect, senior machine learning engineer and the most recent one as an AI/ML product owner where he led a team of data scientists and software engineers to build and shape the vision of Ford's AI/ML (Data Science) Platform. Sina has taught different computer sciences grad and undergrad courses at Wayne State University such as Data Mining and Data Structures and Algorithm. Sina holds a PhD degree in Computer Science and Engineering from Wayne State University and BS and MS degrees in Computer Science and Engineering from Michigan State University.
​
Dennis Michael Sawyers is a Cloud Solution Architect at Microsoft, a Data Scientist and an AI Evangelist. He graduated from Carnegie Mellon University in 2015 with a Master’s Degree in Public Policy & Management: Data Analytics. At Carnegie, he led numerous AI & Analytics projects working with clients including the White House Executive Office, the Department of Commerce and an NBA team. He then joined Ford Motor Company as a Data Scientist where he helped them work with giant volumes of connected vehicle data and apply machine learning to their Marketing, Sales and Service division. Now, as a Cloud Solution Architect at Microsoft, Dennis guides customers on their digital transformation by teaching them the ins and outs of Azure Machine Learning Service and Automated ML at scale.
​
​