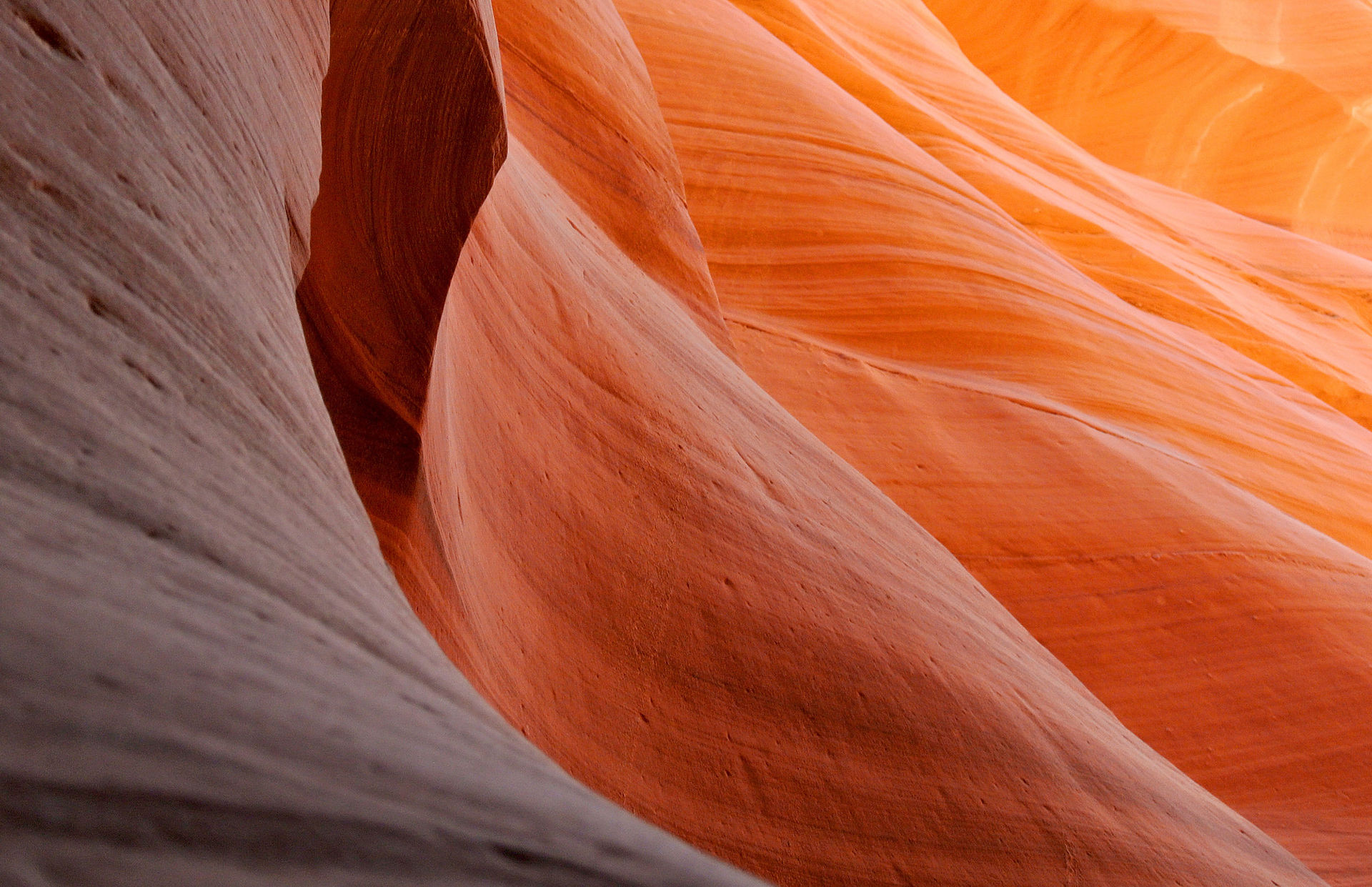

ai4i 2022, AIKE 2022, TransAI 2022
​
Joint Keynotes
(tentative, in alphabetical order)
​
​
George Luger
University of New Mexico, USA
The Foundations for Modern Artificial Intelligence
​
Abstract: We present a brief history of AI research across the more than 70 years since its inception. We begin with an analysis of the mathematical, engineering, psychological, and philosophical foundations enabling modern AI. We then outline and give examples of the three primary research thrusts the discipline has taken over its existence. We conclude offering both important criticisms as well as describing the future promise for current AI research and practice.
​
Bio: George Luger is a Professor Emeritus in the UNM Computer Science Department. His two master's degrees are in pure and applied mathematics from Gonzaga University and the University of Notre Dame. He received his PhD from the University of Pennsylvania in 1973, with a dissertation focusing on the computational modeling of human problem solving performance in the tradition of Allen Newell and Herbert Simon.
​
George Luger had a five-year postdoctoral research appointment at the Department of Artificial Intelligence of the University of Edinburgh in Scotland. In Edinburgh he worked on several early expert systems, participated in the development and testing of the Prolog computer language, and continued his research in the computational modeling of human problem solving performance. At the University of New Mexico, George Luger, a Professor of Computer Science, has also been given a Professorship in the Psychology and Linguistics Departments, reflecting his interdisciplinary research and teaching of Cognitive Science and Computational Linguistics courses and seminars in these areas.
​
George Luger's AI book, Artificial Intelligence: Structures and Strategies for Complex Problem Solving (Addison-Wesley 2008) is now in its sixth edition. Academic Press published his book Cognitive Science: The Science of Intelligent Systems in 1994. His edited collection of readings from the early creators of AI research is presented in his edited collection of papers, Computation and Intelligence, published by AAAI and MIT Press in 1995.
​
Moshe Vardi
Rice University, USA
Machine Learning and Logic: Fast and Slow Thinking
​
Abstract: Computer science seems to be undergoing a paradigm shift. Much of earlier research was conducted in the framework of well-understood formal models. In contrast, some of the hottest trends today shun
formal models and rely on massive data sets and machine learning. A cannonical example of this change is the shift in AI from logic programming to deep learning.
I will argue that the correct metaphore for this development is not paradigm shift, but paradigm expansion. Just as General Relativity augments Newtonian Mechanics, rather than replace it -- we went to the moon, after all, using Newtonian Mechanics -- data-driven computing augments model-driven computing. In the context of Artificial Intelligence, machine learning and logic correspond to the two modes of human thinking: fast thinking and slow thinking.
The challenge today is to integrate the model-driven and data-driven paradigms. I will describe one approach to such an integration --making logic more quantitative.
​
Bio: Moshe Y. Vardi is University Professor and the George Distinguished Service Professor in Computational Engineering at Rice University. He is the recipient of several awards, including the ACM SIGACT Goedel Prize, the ACM Kanellakis Award, the ACM SIGMOD Codd Award, the Knuth Prize, the IEEE Computer Society Goode Award, and the EATCS Distinguished Achievements Award. He is the author and co-author of over 700 papers, as well as two books. He is a Guggenheim Fellows as well as fellow of several societies, and a member of several academies, including the US National Academy of Engineering and National Academy of Science. He holds eight honorary doctorates. He is a Senior Editor of the Communications of the ACM, the premier publication in computing.
​
​
Beverly Woolf
University of Massachusetts Amherst, USA
How are you feeling? Facial Recognition and Other Challenges
Using AI in K12 Education
​
Abstract: AI technology is progressing along many directions and driven by many different organizations. We can’t put the genie back in the bottle; AI is bound to continue to expand. The impact of AI on education will be profound and yet computer-based learning systems will NOT replace human teaching in schools. We need to help the next generation of teachers and students survive in an ethical and reasoned world infused with AI applications and to shape market practices concerning the use of AI in education. We also need to support education leaders who are under continuing pressure to contain teaching costs and move students through schools more quickly.
This talk describes adaptive learning and facial recognition systems that provide a core part of the insights for using AI in K12 education. AI supports personalized learning, in which students learn at their own pace using techniques that work best for them; it supports interaction with objects that are difficult to engage with in the real world; it provides automated student assessment; and it partners with stakeholders to provide useful data and data-derived instructional insights. This talk describes opportunities to develop computing systems that augment K12 instruction by better understanding human cognition. For example, personalized systems might remind learners of things they forgot, support decision making, and focus attention for distracted students.
Quality education will always require active engagement by human teachers and now this active instruction will include collaboration among teachers and AI platforms and tools. As collaborations between learners and computers becomes more commonplace, what challenges need to be solved? What type of transfer of control between people and AI is most appropriate? What opportunities exist for efficient interleaving of instructional contributions for teachers and technology? This talk will address all these issues.
​
Bio: Dr. Beverly Woolf is a Research Professor at the University of Massachusetts-Amherst who develops intelligent tutors that model student affective and cognitive characteristics. These platforms combine cognitive analysis of learning with AI, network technology and multimedia. They represent the knowledge taught, recognize learners’ skills and behavior, use sensors and machine learning to model student affect, and adjust problems to help individual students. Dr. Woolf has developed tutors that enable students to pass standard exams at a 20% higher rate and one system was used by more than 150,000 students per semester across hundreds of colleges. Dr. Woolf published the book Building Intelligent Interactive Tutors, in addition to 200 articles. She is lead author on the NSF report Roadmap to Education Technology in which forty experts and visionaries identified the next big computing ideas that will define education technology and developed a vision of how technology can incorporate deeper knowledge about human cognition and develop dramatically more effective instructional strategies.
​