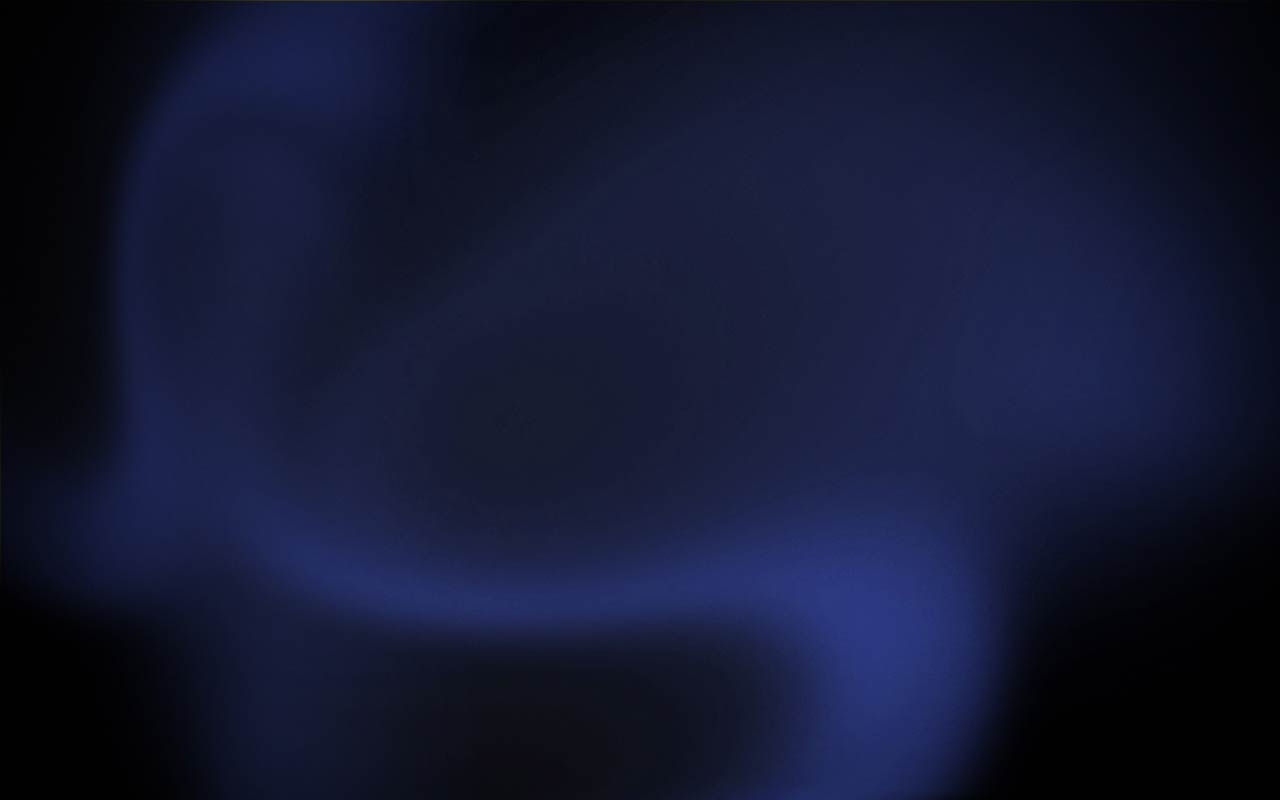

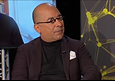

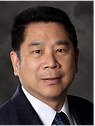
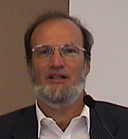
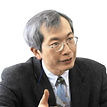

KEYNOTE SPEAKERS
(tentative, in alphabetical order)
Newton Howard
University of Oxford, UK
The Future of Brain and NeuroTech
​
Bio: Dr. Newton Howard is the founder of ni2o, Inc., which is developing a novel, artificial-intelligence driven brain-computer interface to treat a wide range of debilitating neurological disorders and to improve cognitive and athletic performance. His career spans academia, the U.S. military and the private sector. A prolific scientific author and inventor, he is highly skilled in moving research from the lab to military and commercial applications, including technology you may use daily such as wireless hot spots, Google Earth and Google Translate.
​
Dr. Howard holds advanced degrees in Mathematics (Oxford), Cognitive Informatics (Sorbonne), Neurosurgery (Oxford) and a Doctorate of Medical Sciences (Sorbonne). He has made significant contributions to the fields of neuroscience, linguistics and national defense, including the Physics of Cognition (a mathematical framework for modeling complex medical, economic and security equilibriums), Intention Awareness (a theory for predictive modeling of naturalistic systems), Mood State Indicators (an algorithm to model mental processes involved in human speech to predict emotional states), ADAMA (a method of autonomously identifying and contextually understanding metaphors in language), the Functional Code Unit (a method for translating neurological signaling to logical data structures) and most recently, the Brain Code (a multivariate analytical model designed to extract emergent properties of the brain's neuronal system).
​
Dr. Howard is a professor at Oxford and Georgetown. He directs the Computational Neurosciences Lab at Oxford and the Synthetic Intelligence Lab at MIT. He founded and sits on the Board of two nonprofits: the Howard Brain Sciences Foundation, which funds innovative research initiatives to improve our understanding of the human brain and advance the diagnosis and treatment of neurological conditions; and C4ADS, which is dedicated to providing data-driven analysis and evidence-based reporting on global conflict and transnational security issues.
​
​
Giancarlo Guizzardi
Free University of Bolzano-Bozen, Italy
University of Twente, The Netherlands
It's Patterns all the Way Down:
Patterns, Anti-Patterns and Pattern Languages for Next-Generation Semantic Domain Modeling
Abstract: Perhaps the most fundamental notion for successful large-scale collaboratively-built systems is semantic Interoperability. This talk defends the view that to meet the requirements for full semantic interoperability, we need to create an engineering discipline that is based on the followings tenets: (i) real-world semantics should take precedence over pure formal semantics; (ii) real-world semantics is very much about "Ontology" in the true original sense of the term; (iii) before considering automated reasoning and computational non-functional requirements (e.g., computational tractability), semantic representation systems must strive for precision, expressivity, truthfulness to the underlying conceptualization, and pragmatic efficiency; (iv) we need proper foundational theories and engineering (methodological, computational) tools based on these. The talk presents the results of a two-decades research program aimed at addressing these issues. The program is based on two fundamental pillars: the development and use of foundational axiomatic theories (foundational ontologies) and principles in the design of semantic domain modes (domain ontologies, knowledge graphs), and the use of patterns as central complexity management tools in this process. After introducing an Ontology Pattern Language (OPL) for Semantic Domain Modeling that is truly ontology-based, I will discuss Ontological Anti-Patterns (OAPs) as fundamental tools for model validation. Moreover, I will elaborate on the role of having execution semantics of models for model understanding, and validation, and how this approach associated with a particular machine learning technique can support sustainable Anti-Pattern detection and rectification as well as model evolution.
​
Bio: Giancarlo Guizzardi is a Full Professor of Software Science and Evolution at the University of Twente, The Netherlands. He is also a Full Professor of Computer Science at the Free University of Bozen-Bolzano, Italy, where he leads the Conceptual and Cognitive Modeling Research Group (CORE). He has been active for 25 years in the areas of Ontologies and Conceptual Modeling, working with a multi-disciplinary approach that combines results from Computer and Cognitive Science, Logics, Philosophy, and Linguistics. Two well-known results associated to his research program are: the ontologically well-founded version of UML termed OntoUML, which has been adopted by many research, industrial and government institutions worldwide; and the foundational ontology UFO (Unified Foundational Ontology), which has influenced international standardization activities in areas such as Software Engineering and Enterprise Architecture (e.g., the Archimate Standard). He is associate editor for the Applied Ontology, and the Data & Knowledge Engineering journals, as well as member of the advisory board of the International Association for Ontologies and its Applications (IAOA). Over the years, he has conducted many technology-transfer projects in large organizations in sectors such as Telecommunications, Software Engineering, Digital Advertisement, Product Recommendation, Digital Journalism, Complex Media Management, Energy, Tourism, Cybersecurity, Healthcare, among others.
​
C.-C. Jay Kuo
University of Southern California, USA
Deep Learning or Green Learning?
​
Abstract: There has been a rapid development of artificial intelligence and machine learning applications in the last decade. The core lies in a large amount of annotated training data and deep learning networks. There is an emerging concern that deep learning solutions are not friendly to the environment due to their large carbon footprint. As sustainability becomes increasingly important, it is desired to investigate a new learning paradigm that is competitive with deep learning in performance yet with significantly lower carbon footprint.
I have been devoted to green learning since 2014. The technology has become more mature nowadays. Green learning contains quite a few innovative ideas. It has been successfully applied to image classification, point cloud classification, segmentation, registration, texture synthesis, face verification and gender classification, anomaly localization, etc. Performance comparison between deep learning and green learning for several applications will be presented to demonstrate the potential of green learning.
​
Bio: Dr. C.-C. Jay Kuo received his Ph.D. degree from the Massachusetts Institute of Technology in 1987. He is now with the University of Southern California (USC) as William M. Hogue Professor, Distinguished Professor of Electrical and Computer Engineering and Computer Science, and Director of the Media Communications Laboratory. His research interests are in visual computing and communication. He is a Fellow of AAAS, NAI, IEEE and SPIE. Dr. Kuo has received numerous awards for his outstanding research contributions, including the 2010 Electronic Imaging Scientist of the Year Award, the 2010-11 Fulbright-Nokia Distinguished Chair in Information and Communications Technologies, the 2019 IEEE Computer Society Edward J. McCluskey Technical Achievement Award, the 2019 IEEE Signal Processing Society Claude Shannon-Harry Nyquist Technical Achievement Award, the 2020 IEEE TCMC Impact Award, the 72nd annual Technology and Engineering Emmy Award (2020), and the 2021 IEEE Circuits and Systems Society Charles A. Desoer Technical Achievement Award. Dr. Kuo was Editor-in-Chief for the IEEE Transactions on Information Forensics and Security (2012-2014) and the Journal of Visual Communication and Image Representation (1997-2011). He is currently the Editor-in-Chief for the APSIPA Trans. on Signal and Information Processing (2022-2023). He has guided 161 students to their PhD degrees and supervised 31 postdoctoral research fellows.
​
Robert Laurini
INSA Lyon, University of Lyon, France
Semantic Analysis for Geographic Knowledge Modeling
​
Abstract: As it is now common to model business-oriented knowledge based on logic, several hindrances appear when modeling geographic knowledge. Indeed, it is necessary to deal with an efficient representation of space, not only to describe geographic objects, but also their relationships and the rules which can be applied. Starting from a list of geographic rules, this speech will present the semantic aspects of geographic objects, structures, relations which must be integrated without forgetting gazetteers, ontologies, spatio-temporal models (e.g. for floods or pollution diffusion) and external knowledge which could have on impact on local decision-making. Beside semantic aspects, geographic rules must not be encoded not only by means of logic rules, but also using topology and computational geometry (e.g. rule forbidding new construction around 200 m around an historical monument).
​
Bio: Dr. Robert Laurini, presently professor emeritus at the Knowledge Systems Institute, after being distinguished professor at INSA-Lyon, University of Lyon, France, is a well-known specialist in GIS. He was among the first (86-87) to teach GIS at the University of Maryland, USA, at the IUAV University of Venice, Italy, and at several other universities especially in Latin America. He speaks French, English, Italian and Spanish. He has supervised or co-supervised more than 40 PhD's in GIS. His present interests are geographic knowledge, territorial intelligence and digital aspects in Smart Cities. He is vice-president of the Urban Data Management Society whose goal was to promote information systems in municipality, and now in smart cities. He authored or co-authored more than 10 books and more than 250 papers in those domains. He is also the founder of the NGO «Academics Without Borders» which is a network of academic consultants working for the modernization of higher education institutions in developing countries.
See www.laurini.net/robert/ for more details.
​
​
Chung-Sheng Li
Managing Director, PwC Lab, USA
Opportunities and Challenges in Applying Semantic Computing in Finance Operations
​
Abstract: The advances of finance operations, as precipitated by three primary driving forces, are likely to accelerate in the coming future:
-
continued challenges on the need for improved real-time operational visibility and audit quality in a fast evolving business environment,
-
rapid digitization of accounting processes within corporations, especially in the post pandemic world, and
-
advances in semantically interoperable financial reporting (and potentially sustainability reporting) that is conforming to machine interpretable regulations
These trends are closely associated with the trends that businesses are quickly moving their financial data to the cloud, fast maturing of structure reporting (such as XBRL), the adoption of industry ontology, and the focus on fully monetizing the hidden value of data.
In this talk, we will discuss emerging opportunities as we reimagine and apply AI in the future of finance operations from both management and auditing perspectives. This includes using AI to automate (1) connecting the dots within order-to-cash, procure-to-pay, record-to-report, and financial planning and analytic business processes, (2) entity extraction and document comprehension from invoice, purchase order, contracts and lease agreement and how these processes can be transformed by semantic computing (3) evidence reasoning as part of control and substantive testing during an audit procedure, and (4) question answering for finance related questions (5) semantic interoperability among entities in an information value chain. Furthermore, digital transformation of the finance operations also offers the opportunity to refactor the business processes based on common computation in terms of data, process, and policy integrity, resulting in a much more computationally efficient while easily reconfigurable implementation of existing processes.
Bio: Chung-Sheng Li is currently a Managing Director at PwC Lab with the focus on driving AI augmented assurance.. Prior to joining PwC, he was with Accenture Operations as the Global Research Managing Director of AI, with the focus on driving the development of new AI-enabled business process service offerings for Accenture Business Process Services from 2016 to 2019. Previously, he has been with IBM Research between 1990 and 2016 with various technical leadership responsibilities.
His career includes driving research and development initiatives spanning cognitive computing, cloud computing, smarter planet, cybersecurity, and cognitive regulatory compliance. He has authored or coauthored more than 130 patents and 170 journal and conference papers (and received the best paper award from IEEE Transactions on Multimedia in 2003). He is a Fellow of the IEEE.
He received BSEE from National Taiwan University, Taiwan, R.O.C., in 1984, and the MS and Ph.D.degrees in electrical engineering and computer science from the University of California, Berkeley, in 1989 and 1991,respectively.
​
​
Moshe Vardi
Rice University, USA
Machine Learning and Logic: Fast and Slow Thinking
​
Abstract: Computer science seems to be undergoing a paradigm shift. Much of earlier research was conducted in the framework of well-understood formal models. In contrast, some of the hottest trends today shun formal models and rely on massive data sets and machine learning. A canonical example of this change is the shift in AI from logic programming to deep learning. I will argue that the correct metaphor for this development is not paradigm shift, but paradigm expansion. Just as General Relativity augments Newtonian Mechanics, rather than replace it -- we went to the moon, after all, using Newtonian Mechanics -- data-driven computing augments model-driven computing. In the context of Artificial Intelligence, machine learning and logic correspond to the two modes of human thinking: fast thinking and slow thinking. The challenge today is to integrate the model-driven and data-driven paradigms. I will describe one approach to such an integration -- making logic more quantitative.
Bio: Moshe Y. Vardi is a University Professor and the George Distinguished Service Professor in Computational Engineering at Rice University. He is the recipient of three IBM Outstanding Innovation Awards, the ACM SIGACT Goedel Prize, the ACM Kanellakis Award, the ACM SIGMOD Codd Award, the Blaise Pascal Medal, the IEEE Computer Society Goode Award, the EATCS Distinguished Achievements Award, the Southeastern Universities Research Association's Distinguished Scientist Award, the ACM SIGLOG Church Award, the Knuth Prize, the ACM Allen Newell Award, and IEEE Norbert Wiener Award for Social and Professional Responsibility. He is the author and co-author of over 700 papers, as well as two books: Reasoning about Knowledge and Finite Model Theory and Its Applications. He is a Fellow of the American Association for the Advancement of Science, the American Mathematical Society, the Asia-Pacific Artificial Intelligence Association, the Association for the Advancement of Artificial Intelligence, the Association for Computing Machinery, the European Association for Theoretical Computer Science, the Institute for Electrical and Electronic Engineers, and the Society for Industrial and Applied Mathematics. He is a member of the US National Academy of Engineering and National Academy of Science, the American Academy of Arts and Science, the European Academy of Science, and Academia Europaea. He holds seven honorary doctorates. He is currently a Senior Editor of the Communications of the ACM, after having served for a decade as Editor-in-Chief.
​
​